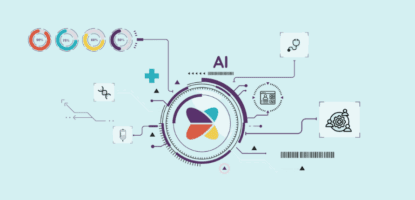
Why You Need AI Clinical Documentation Integrity Software
This is the final part of a 3-part blog series on why traditional clinical documentation integrity (CDI) solutions...
Keep up with the latest insights from experts in healthcare revenue cycle management.
This is the final part of a 3-part blog series on why traditional clinical documentation integrity (CDI) solutions...
Clinical documentation is no longer just administrative overhead—it’s a strategic engine that drives hospital performance. From reimbursement to...
This year’s HFMA Annual Conference provided a clear overview of the challenges and opportunities for health systems in...
Despite more than a decade of regulatory evolution, one rule continues to test the agility and alignment of...
This is part 2 of a 3-part blog series on why traditional clinical documentation integrity (CDI) solutions can...
What is Clinical Documentation Integrity and Improvement? From boosting patient safety to ensuring proper reimbursement, clinical documentation integrity...
Contact us today to schedule a demo or learn more about our AI-powered solutions.